Brief
An a/b test was carried out on a financial services virtual assistant with two opening utterances: one that did not disclose the user was talking to a virtual assistant; and another with a stylised dial tone that stated the bot was a virtual assistant. For 'virtual assistant' calls, we found KPIs roughly stayed the same as 'undisclosed' calls, however, calls were shorter and customer satisfaction survey results improved.
Background
When joining PolyAI, I found a strong consensus that voice assistants should be indistinguishable from a human at the start of the conversation in order to generate greater user engagement. I wanted to challenge this assumption and so organised a design team hackathon to explore this idea. I ran ideation sessions with the team, mapping out how this approach has caused UX degradations in live calls and digging into linguistic theories on how speakers interact with one another in a customer service content.
In reviewing user interactions, we identified three categories of users:
Group 1:
Users that provide a short yet informative utterance. This was the optimal level of information and utterance length, where our natural language understanding (NLU) could suitably extract an intent and direct the user to the correct content.
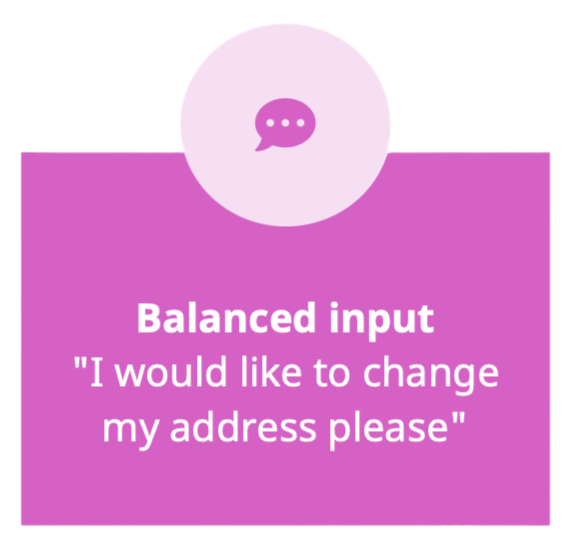
Group 2:
Users that speak in short utterances. These users would often be trying to 'guess' what the system wanted to hear, rather than using natural language. As such, bot performance would falter as not enough useful information would be extracted.
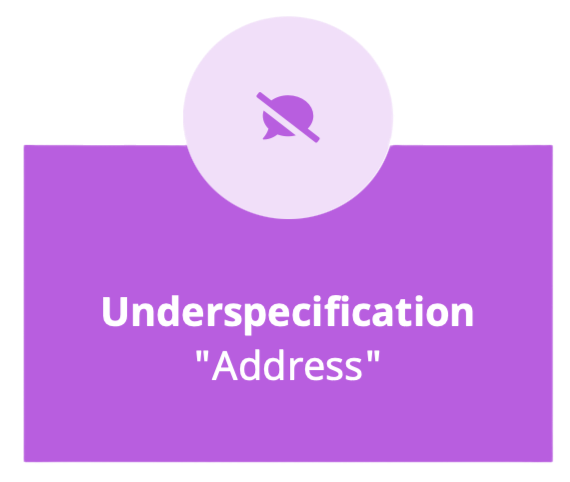
Group 3:
Users that think they are talking to a human and so provide too much information. This level of information would confuse the NLU models, causing too many intents to fire in different combinations, resulting in weaker performance.
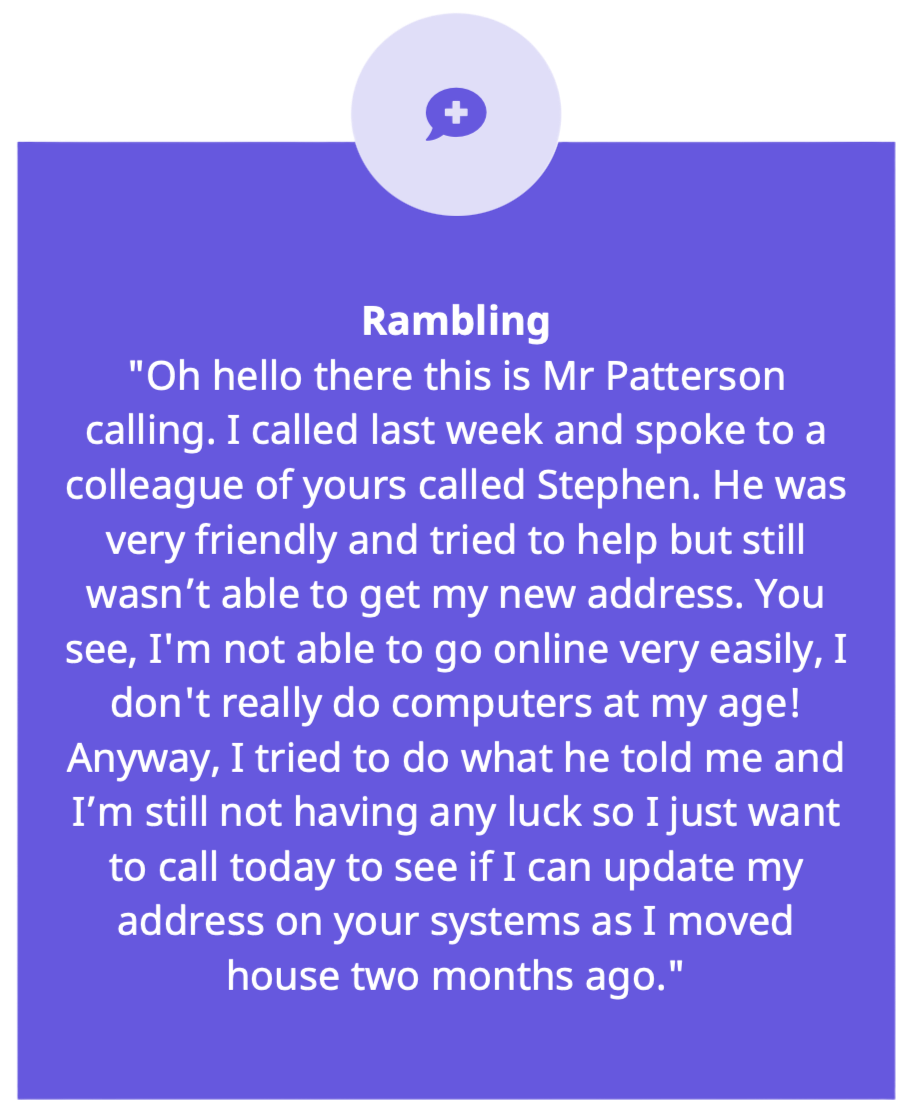
Our hypothesis was that, if we were able to convey to users that they weren’t talking to another human but were talking to something more advanced than a low-quality automated phone line, we could reduce the number of group 2 and 3 users. In short, this would mean we could elicit more informative, succinct utterances from users and, in turn, more efficient and useful interactions.
Method
After discussing the approach with the team, I ran an a/b test on one of my projects — a virtual assistant for a financial services client with an older user population.
The a/b test varied what was said by the bot as soon as the user was connected, known as the 'greet utterance'.
The original greet utterance of the bot stated “Hi, thanks for calling {name of client}. How can I help?”. This utterance did not disclose that the system was a virtual assistant, meaning that more users could assume it was a human. This was used as the ‘a’ utterance.
Caption: a phone dial tone followed by a British male speaker saying "Hi, thanks for calling {a tone masking the name of the client}. How can I help?".
The ‘b’ utterance had two key changes to it. While it discloses the bot is a virtual assistant and its purpose, the dial tone also merges into a piano tone.
Caption: a phone dial tone starts and then, underneath the dial tone, piano chords can be heard before a British male speaker says "Hi, thanks for calling {a tone masking the name of the client}. I'm a virtual assistant, here to help you with all your questions. How can I help?".
The piano sound, while a little cheesy, was chosen for two main reasons.
Firstly, I wanted to surprise the user. It was assumed that, typically, callers think of the dial tone as a separate part of the phone call to the following conversation. It is a technical element that acts as a precursor to being connected to a human: you hear a dial tone, then you are connected. By merging a different sound in with this tone, we would be signalling that something is different about the experience compared to a traditional IVR phone line. As a result, users may be less likely to associate any negative automated experiences they have had and, in turn, be more willing to engage with our system.
Secondly, the piano was chosen due to user demographics, with the majority of users callers being in older age groups. The piano offered a calming tone and was in line with other services for older user bases, identified through market research.
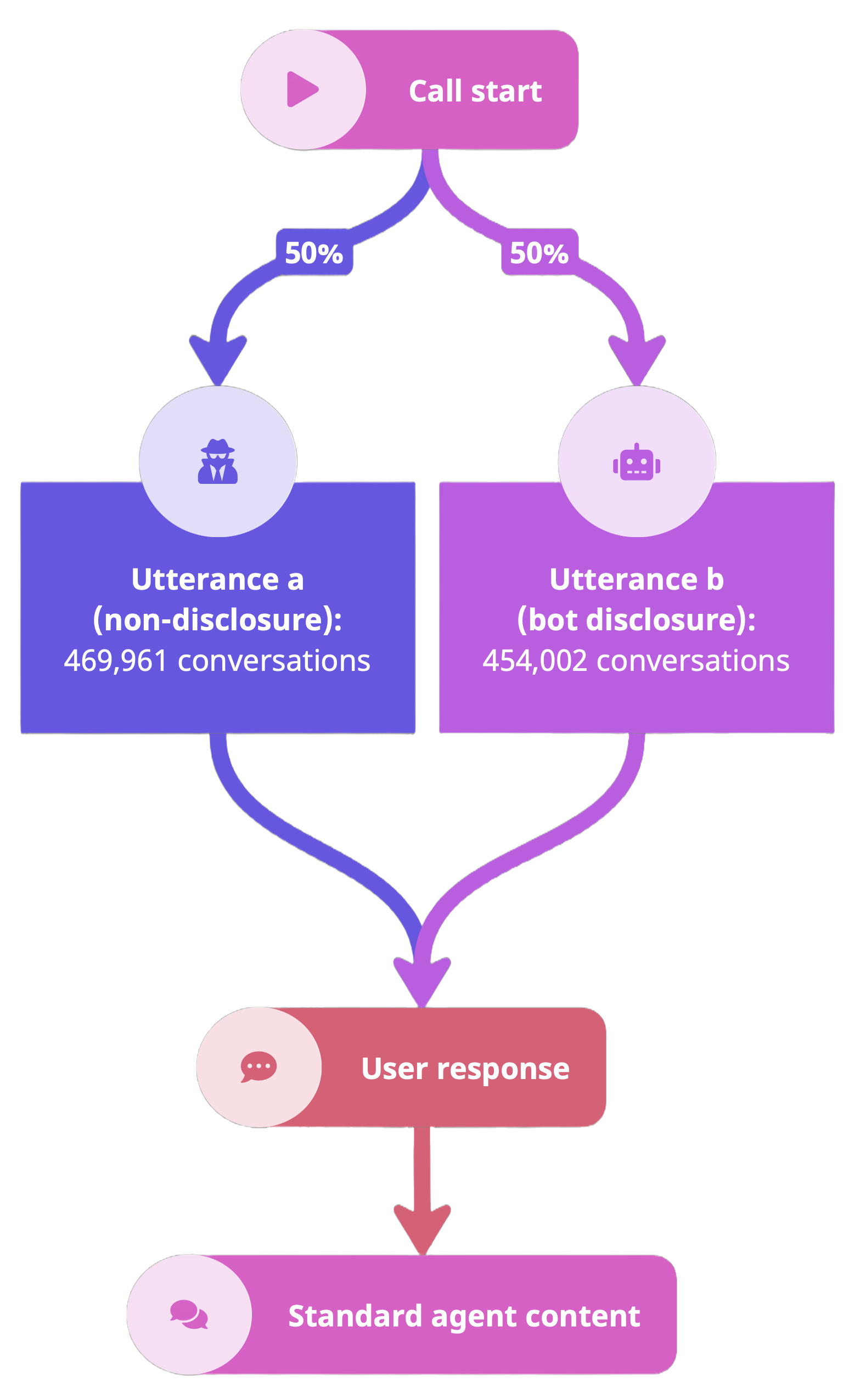
For the test, 50% of incoming calls were routed to utterance a and 50% to utterance b.
Overall, 469,961 calls were routed to utterance a (non-disclosure) and 454,002 calls were routed to utterance b (virtual assistant disclosure).
Results
In summary, KPIs of utterance b calls were either the same or improved compared to utterance a. The user experience in utterance b calls was also improved.
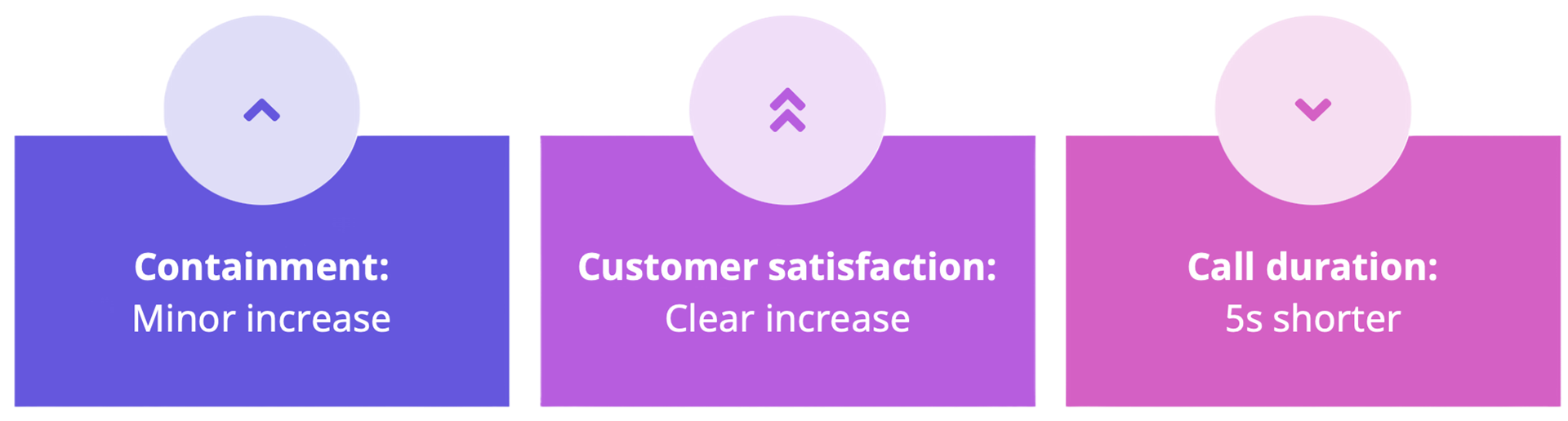
'Containment' (the percent of callers whose queries were fully handled without the need to human input) was higher in version b by one percentage point – a small but consistent increase.
Customer satisfaction in version b was higher than that of a by 3 percentage points, with more users also saying they would use it again. Users who said they were able to complete what they wanted to do also rose by 3 percentage points.
This improvement in user experience was also seen in qualitative call reviews, as users generally gave shorter yet natural utterances and, as a result, interactions were smoother and more efficient – with version b calls being on average 5 seconds shorter than a.
While the test had limitations and measured two experiences rather than individual variables, it was influential in PolyAI's design team thinking and allowed us to build better experiences for end users, with data to back up our design decisions.